What Is Big Data Analytics: 5 Key Benefits Explored
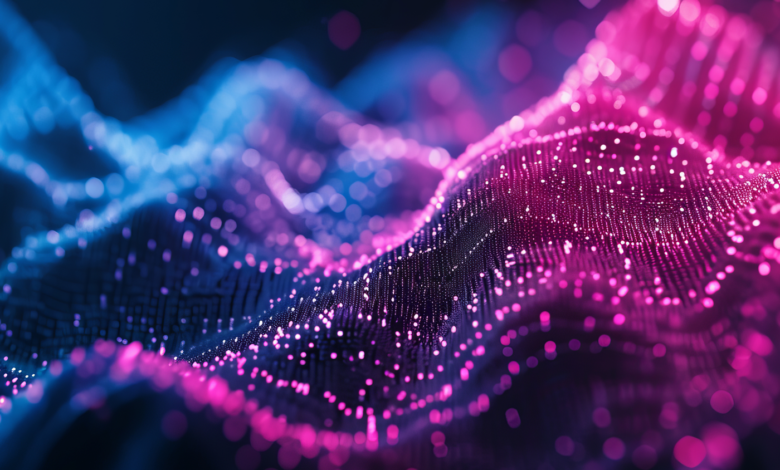
Big data analytics is the process of analyzing and interpreting big and complicated datasets to discover important insights, patterns, correlations, and trends. Advanced technology, algorithms, and statistical models are used to analyze vast amounts of both structured and unstructured data. The fundamental goal is to extract useful information from data, allowing organizations to make better decisions, optimize operations, and gain a competitive edge.
While big data analytics shares the basic premise and functions of other types of data analytics, it is differentiated primarily by the massive scales of the datasets, which require more specialized tools and approaches.
Featured Partners: Data Analysis Software
How Big Data Analytics Works
Big data analytics involves processing large amounts of data to identify patterns, sequences, and correlations that facilitate data-based business decision-making.
Data Collection
The first phase of big data analytics is the thorough collection of data from a wide range of sources, including consumer interactions, financial transactions, social media engagements, and sensor-generated information. This heterogeneous dataset may include structured data (which is rigorously arranged), semi-structured data (expressed in formats such as XML or JSON), and unstructured data (such as raw textual content or photographs). The acquired data is subsequently stored in highly scalable and distributed storage systems.
Data Processing
Sophisticated computing techniques such as distributed computing and parallel processing are used to efficiently handle massive datasets by performing algorithms that convert raw data into a more structured and ordered format appropriate for analysis. This process involves manipulating, aggregating, and preparing data for future investigation. This phase guarantees that the ensuing analysis uses streamlined and optimized data to generate valuable insights.
Data Cleaning
Data cleaning is an important part of the big data analytics process, since it directly affects the dependability and correctness of future studies. Inconsistencies are identified and corrected, missing values are restored, and any data quality concerns that may jeopardize the dataset’s integrity are addressed. Thorough data cleansing improves the trustworthiness of insights produced from the analytics process, instilling confidence in decision-making processes based on the results.
Data Analytics
Finally, the analyzed data is thoroughly examined using modern analytics tools, statistical models, and machine learning (ML) algorithms. These complex tools dive into the dataset to uncover detailed patterns, sequences, and relationships that typical analytical methods may not reveal. Descriptive analytics summarizes previous data trends, whereas predictive analytics predicts future outcomes based on the patterns found. The result is a plethora of insights that enable firms to make data-driven choices, streamline operations, and achieve a competitive advantage in their respective sectors.
Why Big Data Analytics Is Important
The incorporation of cost savings, quicker, better choices, and the development and marketing of new goods and services into big data analytics emphasizes its multidimensional influence on organizational performance. These components work together to provide a comprehensive and strategic approach to exploiting data, improving operational efficiency, and promoting innovation across several aspects of corporate operations.
Cutting Costs
Big data analytics can help organizations detect inefficiencies, enhance resource use, and simplify operations by analyzing large datasets. This rigorous study not only results in operational cost reductions, but also reveals prospective areas for strategic cost-cutting. The result is a leaner and more cost-effective operating model, improving the organization’s overall financial health.
Making Faster and Better Decisions
The ability to make timely and well-informed decisions is critical to success. Big data analytics makes this possible by giving real-time insights gleaned from dynamic datasets. Decision-makers may use this data to adapt quickly to market shifts, new trends, and changing customer expectations. The speed and accuracy provided by big data analytics allow enterprises to negotiate the intricacies of the business landscape with agility, ensuring that decisions are not just timely but also data-driven.
Creating and Marketing New Products and Services
Big data analytics can act as a catalyst for the creation and promotion of new goods and services by allowing organizations to detect market gaps, analyze changing customer preferences, and locate diversification potentials. This knowledge is useful in developing creative products and services that appeal to the target population. The data-driven approach improves the efficacy of marketing efforts by ensuring that new goods are not only well-conceived but also strategically positioned for market success.
4 Types of Big Data Analytics
Four types of analytics operate together in a continuous loop, establishing the framework, investigating the causes of occurrences, forecasting the future, and offering a path for what steps to take.
Descriptive Analytics
Descriptive analytics is the foundation of data analysis, giving organizations a retrospective picture of their activities. This sort of analytics uses statistical metrics and data visualization tools to summarize historical data, offering insights into past performance and patterns. For example, in the retail industry, descriptive analytics may be used to study sales data from various locations and product categories, allowing organizations to find patterns and make informed decisions based on previous sales trends.
Diagnostic Analytics
Diagnostic analytics goes beyond just documenting previous occurrences; it dives into the underlying reasons, addressing the critical question, “Why did it happen?” Techniques like root cause analysis and correlation analysis let companies identify the elements driving certain results. In manufacturing, diagnostic analytics might be used to explore a drop in production efficiency by evaluating factors such as machine downtime, assisting in identifying and addressing the main causes of operational difficulties.
Predictive Analytics
Predictive analytics focuses on the future, using historical data and powerful algorithms to create informed forecasts. Organizations may predict future trends and occurrences by using machine learning and statistical modeling. In addition, predictive analytics is critical in e-commerce for projecting customer demand for specific items, allowing firms to improve inventory management, simplify supply chains, and respond proactively to market demands.
Prescriptive Analytics
Prescriptive analytics is the peak of data-driven decision-making, offering actionable recommendations or hypotheses to attain the desired results. Organizations can use optimization algorithms and simulation models to identify the most successful courses of action. For example, in healthcare, prescriptive analytics may drive individualized treatment plans by assessing a patient’s medical history, genetic information, and current health state, ensuring that healthcare personnel give the most effective and tailored care to each patient.
Advantages of Big Data Analytics
Advantages of big data analytics include increased decision-making and operational efficiency, as well as a range of other benefits:
- Informed Decision-Making—Companies make better decisions by extracting valuable insights from big and diverse information.
- Operational Efficiency—Businesses may enhance operational efficiency by detecting bottlenecks, streamlining operations, and reducing resource waste via data analysis.
- Enhanced Consumer Experience—Understanding consumer behavior and preferences enables businesses to provide more tailored experiences, increasing customer happiness and loyalty.
- Innovation—Big data analytics fosters innovation by offering important insights into market patterns, allowing businesses to uncover potential for new goods or services.
- Competitive Advantage—Organizations may gain a competitive advantage by leveraging data analytics to keep ahead of market trends, respond to consumer requirements more quickly, and adapt to changing business environments.
Disadvantages of Big Data Analytics
The drawbacks of a big data analytics program can include high expenditures and an increased need for specialized expertise, among other disadvantages:
- Data Security Concerns—The sheer amount and sensitivity of data provides security risks; organizations must put in place strong security measures to prevent data breaches and unauthorized access.
- Costs—Creating and sustaining a big data analytics infrastructure may be expensive; costs include technological expenditures, the hiring of experienced people, and continuous upkeep.
- Privacy Worries—Collecting massive volumes of personal data raises privacy concerns; organizations must traverse regulatory frameworks and implement clear data usage rules.
- Complexity and Skill Gap—Managing and understanding data on this scale necessitates specialist knowledge; with a lack of data science specialists across the industry, firms may struggle to attract and keep personnel with the required skills.
- Integration Challenges—Integrating big data analytics into current systems can pose challenges, and organizations seeking to use these technologies face problems due to compatibility concerns and the necessity for seamless connection with legacy systems.
- Overemphasis on Quantitative Data—Relying entirely on quantitative data may leave out qualitative decision-making components, resulting in a limited view of complicated topics.
Big Data Analytics Use Cases
Many organizations increasingly use big data analytics to prepare for the future. Here are some instances of how big data analytics influences strategic planning and decision-making:
- Customer Acquisition/Retention—Insights about consumer behavior and satisfaction lead to improved marketing and engagement strategies for recruiting new customers and maintaining existing ones.
- Customer Segmentation/Targeted Ads—Businesses optimize resource allocation and campaign impact by tailoring marketing efforts to specific client segments based on demographics and interests.
- Product Development—Data insights, such as consumer feedback and industry trends, help firms innovate and produce new goods or improve existing services to remain competitive.
- Price Optimization—Analyzing market trends, rival pricing, and consumer willingness to pay helps optimize pricing strategies for competitiveness and profit.
- Supply Chain/Channel Analytics—Beyond supply chain optimization, data analytics assists firms in analyzing and optimizing distribution networks, resulting in efficient and cost-effective product delivery.
- Risk Management—Data-driven models based on historical data help firms to anticipate and proactively manage possible risks, which is especially important in areas such as banking for making sound investment decisions.
- Improved Decision-Making—Data-driven insights improve decision-making across all company processes, lowering dependence on intuition and promoting informed decisions for overall organizational success.
Big Data Analysis Techniques for Your Organization
Organizations use different kinds of big data analytic techniques to gain insights from huge data sets. This diversified toolkit enables organizations to make informed choices and promote innovation.
Association Rule Learning
Association rule learning is a sophisticated data mining approach to identify significant relationships and patterns in large datasets. One significant use is market basket analysis, which discovers relationships between commonly purchased items. For example, while shopping for spices, association rule learning exposes the ordered grouping of salt, pepper, chile, and other spices in the same section, making the shopping experience easier by showing comparable goods together.
Classification Tree Analysis
Classification tree analysis uses decision trees, which are schematic structures reflecting judgments or tests on characteristics, the outcomes of these tests, and the given class labels. One example is spam detection, which uses a decision tree to assess if an email is spam or authentic. The hierarchical structure simplifies difficult decisions into smaller steps.
Genetic algorithms
Natural selection-inspired genetic algorithms use mutation, crossover, and selection to optimize solutions. The applications span from parameter adjustment to tackling difficult optimization issues—for example, modifying algorithms to improve performance and address complex computational difficulties.
Machine Learning
Machine Learning is a vast area that encompasses several approaches and algorithms that allow systems to understand patterns and make predictions or judgments without explicit programming. This method has several applications in disciplines such image and audio recognition, natural language processing, recommendation systems, and more. Machine learning improves the flexibility and efficiency of processes across several domains by allowing computers to learn from data.
Regression Analysis
Regression analysis is a statistical tool for modeling and analyzing the relationship between a dependent variable and one or more independent variables. This approach is especially beneficial for forecasting numerical outcomes, such as sales projections that take into account various contributing elements. It gives information on how different variables affect the desired output.
Sentiment Analysis
Sentiment Analysis measures sentiment or emotion in text data. This approach is widely used in social media monitoring, customer feedback analysis, and market research to measure public sentiment. Understanding the feelings represented in textual data allows organizations to change their tactics, improve customer happiness, and make educated decisions based on public opinion.
Social Network Analysis
Social network analysis is the study of links and interactions within networks. It recognizes influential nodes and analyzes information flow. For example, in social media or organizational structures, it gives insights into connection dynamics, which aids decision-making and strategy formulation.
Bottom Line: Big Data Analytics is Essential
Big data analytics should be an essential component of the overall data management program for organizations of all sizes. It can provide revolutionary benefits and change established operating perspectives. Regardless of organizational size, incorporating big data analytics enables informed decision-making, optimizes processes, and improves the entire customer experience.
The scalability and flexibility of big data solutions, together with the insights gained, give a competitive advantage while encouraging innovation and agility. In other words, adopting big data analytics is a strategic necessity that sets the path for long-term development, operational efficiency, and a competitive advantage in an ever-changing data-centric world.
If you’re learning about big data analytics, read our buyer’s guide to the 10 Best Big Data Analytics Tools for Business Intelligence Teams in 2024 to find the best software for this essential task.