When Evaluating Travel and Hospitality Artificial Intelligence (AI) Solutions, Look to the Mousetrap
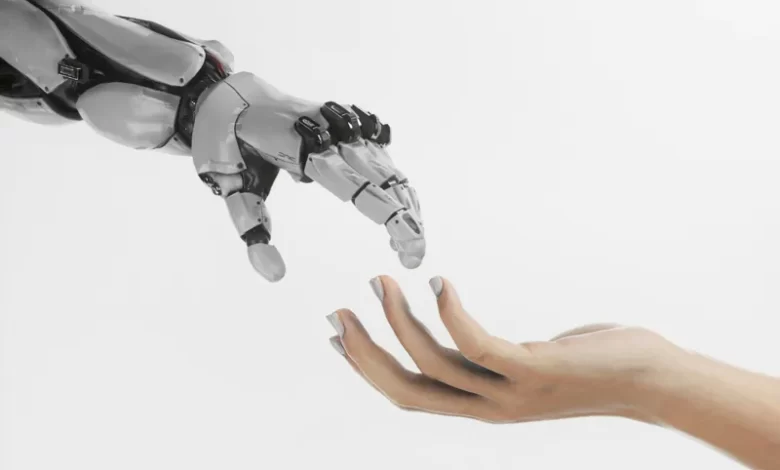
The explosion of new AI capabilities is grabbing headlines, but in a business context, data quality will be the limiting factor in value creation
To catch a mouse, you need more than just a mousetrap – you need the right bait. The trap might be loaded with features – a strong spring, a heavy bar, a sturdy platform (yes, I looked up the parts of a mousetrap for this) – but if the cheese doesn’t entice the mouse, those features have no utility.
While innovative tools are competing for your attention, the quality of data they rely on is equally crucial in delivering true value.
The most popular AI products today are built on public data. LLMs and other models are essentially using the data of the internet to solve all sorts of problems. If there’s a repository of Psych 101 exams and answer keys somewhere, AI can do your homework for you. A trove of corporate headshot images? AI can make your candid photo worthy of LinkedIn.
These types of public use cases seem to dominate the perception of, and discussion around, what AI is capable of delivering. However, when you’re choosing software to solve problems for your business, recognize that those problems are rarely public. The data any software needs to completely, precisely and accurately solve your business problems is simply not available on the public internet.
Imagine that you operate a hotel and you’re able to integrate your favourite BI tool with all the public data of the internet. Admittedly, that would be pretty fun. And we could imagine it solving some interesting problems with all that data.
But would this BI tool help you identify the source of a dip in your hotel’s revenue? No.
Could it even tell you that your hotel’s revenue dipped at all? No.
AI with limited data is no different from BI with limited data. It can’t tell you if your hotel is suffering, why your hotel is suffering, or give you any specific recommendations on how to fix it.
Why not? Because the answer lies in data that is both proprietary and particular.
Proprietary – the data containing the answer is not publicly available.
It is only freely accessible to its owner and the data creator can’t licence it in its original, detailed form. In the hotel example, the owner of the data would be the hotel organisation and the creator of the data would be the Property Management System that manages all the reservations. Nobody outside of the hotel organisation has free access to the data without explicit permission.
Particular – the data dimensions and definitions are particular to an organization
One of many possible dimensions for finding the root cause of a dip in Revenue would be to look at the market segmentation of your guests. While there might be commonalities in market segmentation across hotel operators, they can’t be reliably expected to be the same. A generic concept of market segmentation can’t solve the problems of a particular hotel.
There is another reason I recommend looking beyond the features when evaluating whether an AI solution is a fit for your business: in a business context, expectations for precision and accuracy are heightened.
Many public use cases are fun and low stakes, allowing for some leeway around mistakes. If I miss a question on my psych homework because of a hallucination, I can live with a B instead of an A. (Yes, AI hallucinates.) After all, I used the product to save me time, not make me look perfect.
But giving a bad recommendation to a client can have a negative tangible financial impact and cost someone their job, reputation, and more. And it’s not just accuracy, but precision.
For many use cases, directionally correct is not good enough. Depending on the specific problem and industry, pennies matter. (While outside my particular experience, it’s easy to imagine these expectations being multiplied exponentially in contexts like healthcare, law enforcement, or legal.)
I am not saying these tools are invalid in the business context. My belief is quite to the contrary. I am only highlighting that much of the hard work to ensure they deliver on their promise is the same hard work required to make a BI tool, ML models, or any integrated, SaaS product deliver on its promise: crafting a high-quality dataset. To flip an old adage: quality in, quality out.
So, what makes a high-quality data set?
-
Breadth – includes the variety of data necessary to explore a problem from every critical dimension.
-
Depth – includes the fine-grained detail necessary to form specific conclusions or provide actionable recommendations.
-
Accuracy – represents reality well enough to deliver correct, reliable answers.
-
Consistency – is captured with the same methodology over time to deliver logical, expected results.
-
Timeliness – is updated often enough to deliver relevant outcomes.
-
Impartiality – avoids biases that can skew conclusions.
“AI with limited data is no different from BI with limited data. It can’t tell you if your hotel is suffering, why your hotel is suffering, or give you any specific recommendations on how to fix it.”
I’ll leave you with some practical advice to use when evaluating an AI product’s potential value to your business. Ask the solution provider the following questions:
-
What proprietary and particular data does your product need to give me good answers?
-
Do you source all of the data required to give me good answers? Or will that fall to me?
-
Is the data you source high enough quality to give accurate, precise, and complete answers? Use the list above to ask detailed questions for each dimension of data quality.
-
How do you ensure data quality? How rigorous is that process? Who is involved and what tools are involved? What is my role in ensuring data quality?
Of course, evaluate the mousetrap. But please … don’t forget about the cheese.
Gregory Peppel
Head of Business Intelligence Products – For over 15 years, Gregory has specialized in hospitality-centered product development, creating vertically-integrated SaaS BI products and cultivated high-performance product development teams. His background includes a successful tenure at Avero, where he contributed to the expansion of their F&B BI product into twelve of the top fifteen largest global hotel brands. Connect with Gregory on LinkedIn.
About Lighthouse
Lighthouse (formerly OTA Insight) is the leading commercial platform for the travel & hospitality industry. We transform complexity into confidence by providing actionable market insights, business intelligence, and pricing tools that maximize revenue growth. We continually innovate to deliver the best platform for hospitality professionals to price more effectively, measure performance more efficiently, and understand the market in new ways.
Trusted by over 65,000 hotels in 185 countries, Lighthouse is the only solution that provides real-time hotel and short-term rental data in a single platform. We strive to deliver the best possible experience with unmatched customer service. We consider our clients as true partners—their success is our success.