Will AI Outgrow Its Resource Shoes?
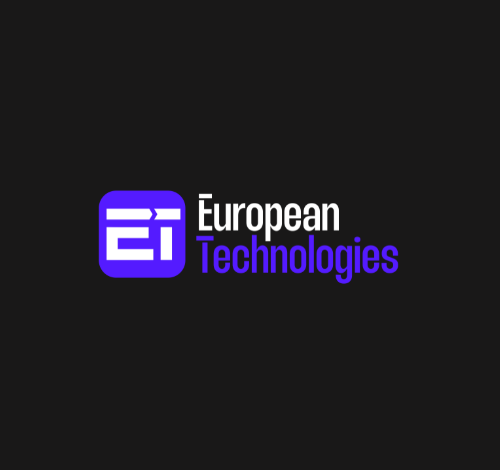
The Gist
- Industry bottleneck. LLM expansion challenges intensify with increased data and compute requirements.
- Hardware evolution. Custom AI chips are crucial for next-generation model training and efficiency.
- Synthetic shift. Utilizing synthetic data is becoming a key strategy in training more capable AI models.
Each new generation of large language model consumes a staggering amount of resources.
Meta, for instance, trained its new Llama 3 models with about 10 times more data and 100 times more compute than Llama 2. Amid a chip shortage, it used two 24,000 GPU clusters, with each chip running around the price of a luxury car. It employed so much data in its AI work, it considered buying the publishing house Simon & Schuster to find more.
Afterward, even its executives wondered aloud if the pace was sustainable.
“It is unclear whether we need to continue scaling or whether we need more innovation on post-training,” Ahmad Al-Dahle, Meta’s VP of generative AI, told me in an interview. “Is the infrastructure investment unsustainable over the long run? I don’t think we know.”
Related Article: What Are Large Language Models (LLMs)? Definition, Types & Uses
Meta Faces Limits in LLM Expansion
For Meta — and its counterparts running large language models — the question of whether throwing more data, compute, and energy at the problem will lead to further scale looms large. Since LLMs entered the popular imagination, the best path to exponential improvement seemed to be combining these ingredients and allowing the magic to happen. But with the top bound of all three potentially in sight, the industry will need newer techniques, more efficient training and custom built hardware to progress. Without advances in these areas, LLMs may indeed hit a wall.
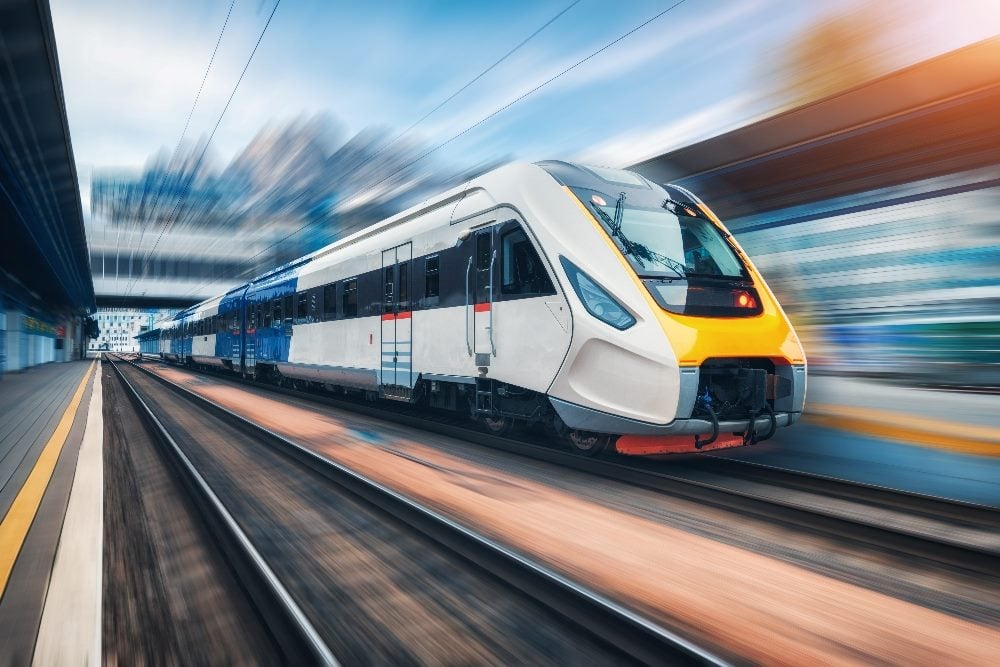
Related Article: Did Meta Just Top ChatGPT With Its Release of Llama 3’s Meta AI?
New Architectures to Revolutionize LLM Scaling
The path of continued scale probably starts with better methods to train and run LLMs, some of which is already in motion. “We are starting to see new kinds of architectures that are going to change how these models scale in the future,” Swami Sivasubramanian, VP of AI and data at Amazon Web Services, told me in an interview.
Sivasubramanian said researchers within Stanford and elsewhere are getting models to learn faster, with the same amount of data, and 10 times cheaper inference. “I’m actually very optimistic about the future when it comes to novel model architectures, which has the potential to disrupt the space,” he said.
Already, new methods of training these models seem to be paying off. “The smallest Llama 3 is basically as powerful as the the biggest Llama 2,” Mark Zuckerberg said on the Dwarkesh Patel podcast the week of the Llama 3 release.
Related Article: Looking Under the Hood at Generative AI History
Synthetic Data Boosts AI Model Training
To fuel these models — and get around potential bottlenecks in exhausting real world data — synthetic data created by AI is playing a key role. Though not fully proven yet, this data already made its way into model training. “Our coding abilities on Llama 3 is exceptionally high,” Meta’s Al-Dahle said. “Part of that was really being innovative and pushing on our ability to leverage models to generate synthetic data.”
AI Chips Propel LLM Advancements
Along with finding better models, LLM progress likely depends on building better chips that can train and run these models faster and more efficiently than traditional chips. While NVIDIA GPUs are exceptionally useful for large language models, they aren’t purpose-built for them. Now some chips built specifically for generative AI are showing promise. Researchers like Andrew Ng have praised Groq, one buzzy name, as the type of chip that works fast enough to take generative AI to the next level, especially as the field pushes toward agents.
Related Article: Generative AI Technologies: Now and in the Future
Tech Giants Build Speedy AI Chips
Meanwhile, companies like Amazon, Intel, Google, and others are building “accelerators,” or custom chips that can run AI processes fast. At Amazon, Sivasubramanian said, the company’s purpose built Trainium chips are “designed with the sole purpose of being able to train these large language models” and already four times faster than the first generation.
Given the need and the opportunity ahead, it’s no wonder OpenAI CEO Sam Altman is reportedly raising a lot of money to build chips powerful enough to achieve his aims.
Energy Limits Loom Over AI Progress
The one LLM constraint that’s been little discussed is energy, and it may be the most important.
“There’s a capital question of — at what point does it stop being worth it to put the capital in? — but I actually think before we hit that, you’re going to run into energy constraints,” Zuckerberg told Patel. He floated the idea of building a 1 gigawatt datacenter to advance AI, or something approximating a meaningful nuclear power plant. But given regulatory approvals and the build outs complexity, it could take years to produce. “I think it will happen,” he said. “This is only a matter of time.”
Energy Questions Hinder LLM Advances
Until we get to such massive energy allocation, it may be difficult to say how much room LLMs have left to improve. But it seems like sooner or later, we will find out. “I am not thinking about it myself,” Sivasubramanian said with a laugh, of a nuclear-level plant to run AI models, “but I can’t speak to my infra team.”