Wipro applying Data, AI/ML and Generative AI to the Telecom Industry
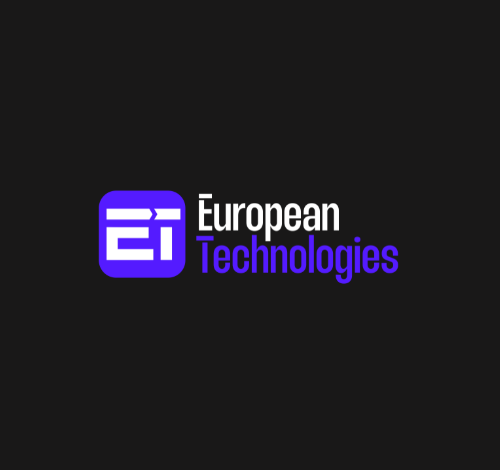
By Vanitha Jayasuriya, Full Stride Cloud Solution Lead, Germany – Wipro
By Yedu Kuruvath, Alliances and Partner Development Lead, AI Practice – Wipro
By Shaban Saddique, AWS Business Group Director, Europe – Wipro
By Benson Philip, Sr Partner Development Manager, EMEA – AWS
By Bindhu Chinnadurai, Sr Partner Solutions Architect, EMEA – AWS
![]() |
Wipro |
![]() |
Introduction
Over the past few years telecom industry has not only transformed its own business, but has also helped accelerate innovation in other smart industries. Technologies like wireless communication has powered applications that run autonomous driving, video streaming, virtual-reality gaming and many more. Wireless communication networks have increasingly become more robust and offer ultra-low latency, reliability and cost-efficient solutions. Customers are able to use this network to transform, digitize, and reimagine consumer experiences. Because this is a continuously evolving industry, there is no shortage of challenges . Cost, workforce culture and meeting sustainability goals rank among the industry’s most urgent imperatives. It is important that the telecom industry responds quicky and effectively to the pressures that customers are facing.
According to TM FORUM, 14 leading CSPs have incorporated autonomous networks into their strategies with the goal to reach level 4 maturity by 2027. Level 4 maturity entails a highly autonomous telecom network that runs with minimal to no human intervention—able to configure, monitor, and maintain itself independently. Telcos have the vision to provide “Zero X” (zero wait, zero touch, zero trouble) experiences for their consumers and vertical industry users. For internal users, the vision is to enable “Self-X” capabilities to self-service, self-fulfillment and self-assurance through intelligent network and autonomous operations.
The amount of data gathered by telecoms is immense. In this blog post, we will discuss on leveraging data to solve challenges that the industry faces. AI/ML (Artificial Intellignce/Machine Learning) can be used to derive real-time insights from this data, including predictive analytics, network optimisation, hyper personalised customer service, fraud management and operational efficiency. Further, the possibilities of generative AI has opened a floodgate of use cases as communication service providers (CSPs) embark on this autonomous transformation journey.
Why AI/ML and generative AI adoption important for telecom
Historically, automation has helped drive cost effectiveness and improve efficiency but the rule-based nature makes it more reactive. Rather it would be effective to inculcate the mechanism of decision making and driving actions from data in a proactive manner. AI/ML and generative AI based analysis during the planning, design and deployment cycles can help CSPs be more proactive. According to a recent Gartner report, by 2026, 95% of communication service providers will deploy data, analytics and AI initiatives to improve their product planning and enhance their customer experience, up from 50% in 2022. AI/ML models can provide insights based on historical data to find patterns and make informed decisions.
AI/ML models can handle more complex business problems as they learn, predict and can decide based on what’s happening on the network. Unlike rule-based anomaly detection, AI/ML systems aren’t bounded by the same lack of context and can accelerate root cause correlation dynamically and derive the next step of action. For example, CSPs can more effectively optimize bandwidth allocation based on user demand and intelligently route and prioritize traffic. Additional insights can also be gained by analysing the data consumption pattern of users. For example, the system might help predict the propensity to buy in certain areas. This could be used to focus upselling direct services or cross-selling auxiliary service for things such as additional cloud storage or OTT subscriptions.
Telecom industry is one of the large contributors of global greenhouse gas emissions. AI/ML can play an important role in enterprises meeting their sustainability commitments by using technology and processes that are low in carbon emissions. For example, by using drones and computer vision to inspect physical infrastructures like telecom towers, which would have been an otherwise labour intensive process involving the movement of people and equipment. AI/ML can help reduce energy consumption in both Radio Active Networks (RAN) and Core. For example, AI/ML models can predict network traffic and adjust shutdown times dynamically. It can extend savings windows compared to static schedules, avoiding any degradation of network performance.
Why Generative AI?
Great use cases for AI/ML, isn’t it? Then why generative AI? What are the areas that telco customers are looking to leverage Generative AI? Many customers have already embarked their journey to improve customer experience with the help of generative AI. Capabilities such as chatbots, virtual assistants, intelligent contact centres, personalization, guided assistance for tech usage, and content moderation are just a few. Today, many enterprises are looking to boost employee productivity. Business users can leverage generative AI powered conversational searches (for example in contractual obligations) and text summarization (for example in contract reviews). Marketing teams can leverage generative AI for content creation such as social media posts for customer engagement. IT teams can improve productivity by leveraging the code generation capability of generative AI in IT and legacy modernization of enterprise systems. Another area is business operations; for example, in network planning and design. Generative AI can help estimate cell load and traffic routing for various scenarios which results in improvements to network coverage and spectrum utilization. With generative AI capabilities, CSPs can also innovate efficiently without negatively impacting the average revenue per user (ARPU), thereby optimizing costs.
AWS continues to play a key role in democratizing AI/ML and Generative AI in making it accessible to anyone who wants to use it, including more than 100,000 customers of all sizes and industries. AWS has the broadest and deepest portfolio of AI and ML services. Each of these layers abstracts the complexity of AI/ML development and enterprises can leverage them based on their maturity and readiness. Amazon SageMaker is the easiest way for all developers to build, train, and deploy models by abstracting away the infrastructure needed to train and host models. Amazon Bedrock is a fully managed service, and is the easiest way to build and scale generative AI applications. Through a single API, it offers a choice of high-performing foundation models (FMs) from leading AI companies that brings a broad set of capabilities needed to build generative AI applications, simplifying development while maintaining privacy and security. That’s the reason telecom customers like T-Mobile, Globe and many more have worked with AWS to help them transform and leverage AI/ML across their value chain.
How does Wipro help our customers embark the transformation journey
Wipro Limited, a leading technology services and consulting company, has announced the launch of Wipro ai360. Wipro ai360 is a comprehensive, AI-first innovation ecosystem that builds on Wipro’s decade-long investments in artificial intelligence (AI). The goal is integrating AI into every platform, every tool, and every solution used internally and offered to customers – with responsible AI at its core. With deep engineering and product design expertise, Wipro partners with communication service providers (CSPs) to take them closer to their 5G ambition, transforming experience for their customers and improving the intelligence quotient of the enterprise.
To ensure that customers are using AI responsibily, Wipro has developed a comprehensive framework called ‘Wipro Enterprise Generative AI Framework’ (WeGA) built on AWS. It is tightly integrated to fundamental building blocks to help operationalize access to LLMs and keeping a tight control on hallucinations, security, safety and traceability.
Wipro is a partner leading Enterprise IQ (E-IQ), an inteligence infused enterprise transformation journey for many telecom enterprises. E-IQ framework serves as a tool to evaluate the current state of an organization and determine its readiness to adopt and implement intelligent solutions. Through this assessment, Wipro identifies areas for improvement and provides recommendations for enhancing the organization’s intelligence quotient. Below are a few usecases that Wipro has helped customers adopt AI/ML and generative AI solutions with the E-IQ framework that have created high impact on customer experience and service assurance.
Use Case #1: Virtual Agent
Wipro is working with customers on contact center solutions that help manage and process large amounts of data, and provide real-time Customer Experience (CX) enhancements. The Large Languages Models (LLMs) built and deployed by Wipro for customer facing virtual agents have the ability to produce human-like interactions. Real-time agent assistance and further post-call summarization is made possible using generative AI on AWS. LLMs are able to make use of historic data like call history, integration with enterprise systems like ERP/CRM and conversational transcripts from active conversations with the caller. They can understand the intention and context of the customer call and recommend appropriate answers to the agent. It can also determine customer sentiment and how it changes over the life of the interaction. This experience has taken conversational AI to the next level.
For the last few years, Wipro has been working with customers on end-to-end solutions on Amazon Connect, AWS’ AI-powered contact center. For those who already have a contact center in place, LLMs developed on Amazon SageMaker Jumpstart and AWS Contact Center Intelligence (CCI) solutions have been deployed. AWS CCI uses a combination of AWS ML-powered services such as Amazon Transcribe, Amazon Comprehend, Amazon Lex, Amazon Kendra to provide self-service virtual agents, real-time call analytics and agent assist, and post-call analytics.
Use Case #2: Social Lens
CSPs today receive a vast amount of feedback and input regarding offerings and processes from their customer base through product reviews, feedback forms, social media and hotline conversations. Manually correlating issues mentioned in this unstructured data with specific features or components within their technology stack that cause these issues is time-consuming and prone to errors. The lack of automated correlation hampers the efficiency of issue resolution, leading to customer dissatisfaction and increased operational costs. Wipro’s generative AI-based solutions are built on Amazon SageMaker along with Amazon S3, Amazon API Gateway and AWS Lambda. Wipro’s solutions aim to efficiently and accurately correlate customer-reported issues with the specific features or components in their technology stack. The solution leverages the power of ML and natural language processing (NLP) to automate the process of identifying, summarizing, classifying and correlating issues to exact technology components. Thereby, providing an early warning system and reducing the operational overhead.
Use Case #3: Network Performance Analysis
Although CSPs have collected a lot of historical data across different geos, one recurring pattern across customers irrespective of their geography is the siloed nature of data. It’s difficult to gain insights from data that is siloed. And CSPs need insights to make informed decisions and actions to improve the network. Of the many factors that can influence, customer trust is deep rooted in network reliability. To provide hyper personalised customer experience, it is essential to understand network health within subscriber context. Smart CSPs requires early attention to network performance especially for services that are related to safety and security of the service provider. CSPs can service customers better by bringing together the power of AI in union with generative AI powered network-ops.
Wipro is working along with CSPs to build generative a AI-based knowledgebase on AWS. It serves as a tool for network operations and a repository where static data such as network topology, KPI definitions and specifications along with the real-time data like user device is stored. Data is ingested into managed databases such as Amazon Neptune, which enables building queries that efficiently navigate highly connected datasets. Embeddings encode this data into vectors that capture the meaning and context of an asset. This allows the LLM to find data by searching for neighbouring data points using Amazon OpenSearch Service. Operations teams can interact with the LLMs built on Amazon Sagemaker Jumpstart and those available on Amazon Bedrock through voice or text. The NLP capability of the LLM model will convert voice questions into a text query dynamically in order to locate and retrieve the correcte data points.
Diagram 1 – Vector datastores for Retrieval-Augmented Generation for Knowledge-Intensive NLP Tasks
The knowledgebase helps with identifying patterns, detecting anomalies, and predicting network failures. This empowers the operations team to make decisions on the fly – for example allocate network bandwidth based on high traffic regions. The on-demand observability feature helps the CSPs in multiple other ways like minimizing systems outages, improving customer experience and saving energy. The knowledge base can ensure that chatbots or any conversational UI (user interface) provide accurate information to the end user and also suggesting the next best action.
Diagram 2 – GenAI-based Knowledge for Operations
It is important that generative AI systems can be trusted to provide accurate information to minimize impact to systems or reputation. To minimize LLM hallucinations, the vector databases complements the LLM by providing additional context. This will include embeddings representing knowledge or data that was not included in the training of the LLM, such as customer proprietary information, up-to-date data sources, bespoke documentation. Wipro has been leveraging AWS offerings for vector database requirements like k-NN(k-Nearest Neighbor) search using Amazon OpenSearch Service. The solutions makes use of pgvector extension to store embeddings from ML models in the database and to perform efficient similarity searches using Amazon RDS for PostgresSQL. Accurate predictions from graph data are made with Amazon Neptune ML that uses Graph Neural Networks (GNNs).
Conclusion
In this post, we discussed the current challenges faced by telcos as they balance complexity and scale of the infrastructure and operations with the desire to innovate on behalf of their customers. We further discussed a few usecases and how Generative AI can help solve those complexities and the potential value that it can deliver for telecom networks and businesses. Finally, we discussed on how Wipro and AWS can support the implementation of generative AI usecases for CSPs by providing discovery and the requisite framework, tools, and services to eventually facilitate innovation.
.
Wipro – AWS Partner Spotlight
Wipro is an AWS Advanced Technology Partner and AWS
Competency Partner Its AI/ML solutions drive enhanced operational efficiency, productivity, and superior customer experience for many enterprise clients.
Contact Partner | Partner Overview | AWS Marketplace