The art of teaching artificial intelligence
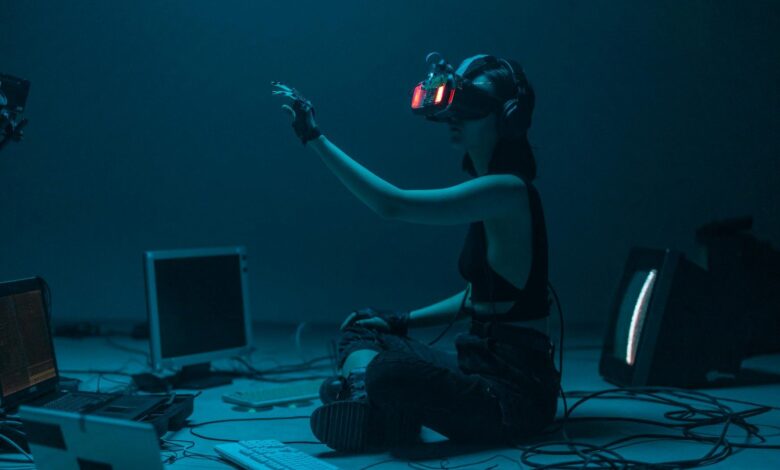
One of its chapters is almost entirely devoted to the work of George Gallup, founder of the famous opinion institute, a pioneer in applying concepts as disparate as anthropology and statistics to audience measurement and the design of polls and surveys, with the aim of becoming familiar with people’s tastes and monitoring the evolution of public opinion.
Derek emphasises one of the revolutionary conclusions reached by Gallup: the distinction between polls to measure the present, and surveys to measure the future, each with its own methodology because “…people are generally very good at expressing their feelings here and now, but are much less reliable when it comes to reporting their habits (especially bad habits) or projecting their future wants and needs”.
In a no less excellent essay, “The Mom Test“, Rob Fitzpatrick shares his extensive experience interviewing potential customers during product ideation processes, and advises us what kind of questions bring us closer to identifying their real needs: “How to have conversations with your customers and validate your business idea when everyone is lying” reads the subtitle.
The story is full of juicy anecdotes, such as this one:
I once had a client who, whenever a certain process in his organisation was named, used emotionally charged terms such as ‘DISASTER’, while shouting and gesturing with his arms. But when I asked him one day what impact that problem had [for his organisation], he shrugged his shoulders and replied “Problem? They gave us more interns and we put them on it. It’s going pretty well now.
As Gallup suspected, human beings’ forte is sharing our emotions; but we don’t always express what we really want, and even when we are clear about our needs, we too often communicate them in ambiguous, rushed, or inadequately prioritised ways. So much so that human conversations, replete with misunderstandings, have elevated the capturing and prioritisation of requirements to an art form.
How to address this human quirk in Digital Transformation projects
We have been talking for many years about digitalisation, automation, robotisation of processes… all of these projects are based on identifying needs, prioritising them and defining requirements. And in the identification of habits, it is becoming less and less strange to bet on techniques made popular by Gallup, such as shadowing (learning by accompanying), safari (learning incognito); or adaptations of these techniques to the analysis of business workflows, such as process mining.
More recently, Artificial Intelligence (AI) has made its way into our everyday conversations, with the veiled promise of solving our needs in an immediate and simple way: “ask the chatbot“. The story works so effectively that more and more organisations with no previous experience in this area are feeling the compelling need to jump on the AI bandwagon.
In a previous article, we explained how formidable challenges faced by classical approaches to automation and process robotisation continue to be faced by Artificial Intelligence.
One of them, as Gallup anticipated almost 100 years ago, lies in defining as precisely as possible our own needs; and it will be the one we focus on today.
In the case of AI, that is precisely one of the roles of a prompts engineer, that of intermediary between the needs of our organisations and Artificial Intelligence. Or, in the words of The Digital Business School, the group of professionals specialising in “…designing questions, commands or phrases that enable AI to be able to generate coherent and useful answers”. If George Gallup were listening, he would be smiling with satisfaction.
Perhaps we can better understand the role of prompt engineering with a couple of examples. Over the last two decades we have become accustomed to interacting with Internet search engines such as Google, Bing or Yahoo. Something as seemingly everyday as an Internet search gives us the first clue that there are Internet users who are more adept at this facet. As Jesús Fernández Villaverde, Professor of Economics at the University of Pennsylvania, reports in an article published in the digital newspaper El Confidencial:
“Twenty years of experience have taught me that an almost perfect predictor of future success of graduate students in their doctoral dissertation is their ability, early in their studies, to find the relevant research articles in a subject area and summarise them fluently. Internet search engines are the same for all students in the freshman class, and while some students can figure out which articles in a research area are important and solid, other students cannot distinguish the wheat from the chaff.
Voice assistants
Another piece of evidence drawn from our daily lives comes from the recent proliferation of voice assistants. Hands up who hasn’t experienced momentary frustration when giving an instruction to Siri, Alexa or Aura without getting the expected result, only to find that another member of our family then rephrases the command and succeeds.
Are we not acting as improvised prompts engineers when we enter a search term or give an instruction to a voice assistant?
And the reality is stubborn: soon after starting to practice, we will discover that you are not born knowing how to whisper to an AI to achieve the result we would like to obtain; not even to refine a first result.
Computational thinking to the rescue
Gallup passed away in 1984, leaving the profession he founded without its leading figurehead. It was 2006 when Jeannette Wing, a professor in the Department of Computer Science at Carnegie Mellon University, first used the term ‘Computational Thinking’ to describe the patterns of reasoning in software engineering and the benefits that this way of thinking could have on all of us.
As our friends at Programamos like to remind us, 2023 will be the 18th anniversary of the publication of that article. The term has been evolving and becoming more nuanced, until reaching a consensus, not unanimous but reasonable, around this possible formulation:
“Computational thinking is the (human) ability to solve problems and express ideas using Computer Science concepts, practices and perspectives“.
The benefits mentioned by Dr. Jeannette Wing are not limited to solving real-life problems, but can also be applied to expressing ideas. With the particularity that, in the field of classical computer science, there is no room for ambiguity: for better or worse, a computer algorithm has a precise result.
A common belief is to equate computational thinking with learning to program. However, I would like to stress that computational thinking does not necessarily imply knowing how to program. In fact, computational thinking helps to tackle everyday tasks, whether it is planning a holiday, organising a party, solving a puzzle or formulating the right questions to diagnose an illness.
What if we invested effort in applying this new paradigm to expressing certain ideas, such as the definition of requirements, or priorities? Would we solve a substantial part of the problems that our organisations face on a daily basis if we reduced the ambiguity in the definition of these, and shortened the time consumed in clarifying what has to be done? Would we multiply the benefits of having the most experienced team of programmers, with the most intuitive to use Zero Code Digitalisation tool, with the best trained Artificial Intelligence Chatbot? Or are we facing yet another passing fad?
Looking at education, computational thinking has recently been incorporated into the LOMLOE curriculum in pre-school, primary and compulsory secondary education. It is up to us to wait patiently for a new generation of professionals to enter the world of work in order to gauge its strengths and weaknesses, or to take the initiative by experimenting with this discipline in the training plans of our organisations.